How to Be Statistical Analyst - Job Description, Skills, and Interview Questions
Increasingly, employers are seeking individuals with advanced skills in statistical analysis to fill positions as Statistical Analysts. This trend is due to the growing complexity of data and the need for businesses to make informed decisions based on detailed analysis of that data. Statistical Analysts use their expertise in mathematics, computer programming, and data manipulation to model data and interpret the results.
They are tasked with providing insights to businesses about trends, correlations, risk, and customer behavior. As a result, businesses are able to make more effective decisions and achieve better outcomes.
Steps How to Become
- Earn a Bachelor's Degree. Most entry-level positions in the field of statistical analysis require at least a bachelor's degree in statistics, mathematics, economics, or another related field.
- Get Relevant Experience. Many employers prefer to hire applicants with experience in the field. Relevant experience can be obtained through internships, research projects, and volunteer work.
- Earn an Advanced Degree. Earning a master's degree or PhD can lead to higher-level positions and higher salaries.
- Acquire Technical Skills. Statistical analysts must be proficient in using statistical software packages such as SAS and SPSS. They must also be knowledgeable about database management systems and be able to create complex mathematical models.
- Obtain Certifications. Professional certifications from organizations such as the American Statistical Association or the Institute for Operations Research and the Management Sciences can help applicants stand out from the competition.
- Network. Establishing strong connections in the field can help applicants find job opportunities and stay up-to-date with industry trends.
As technology continues to advance, it is important for statistical analysts to stay up-to-date and capable in their field. This requires dedication to continuing education and self-learning, as well as staying current on relevant industry news and trends. Keeping up with the latest trends in data analytics and statistical techniques is essential for staying competitive in the job market and being able to provide the most value to employers.
utilizing tools like data visualization and data mining can help give a better understanding of data sets, enabling more accurate predictions and analysis. Finally, staying connected with peers in the statistical field can help provide insight into new methods and trends. By continuously pursuing knowledge and staying connected, statistical analysts can help maintain a competitive edge in their field.
You may want to check Business Consultant, Quantitative Researcher, and Demographic Analyst for alternative.
Job Description
- Data Scientist: Analyzes data to identify patterns and trends in complex datasets, develops predictive models, and implements data-driven solutions.
- Business Intelligence Analyst: Collects and interprets data to inform business decisions and strategies.
- Data Modeler: Develops and maintains data models to represent the structure of an organizations data.
- Database Administrator: Installs, upgrades, and manages databases and database systems.
- Data Architect: Designs, implements, and maintains data solutions that meet organizational standards.
- Statistical Analyst: Gathers, organizes, and analyzes data to draw meaningful conclusions, identify correlations, and formulate hypotheses.
Skills and Competencies to Have
- Advanced knowledge of statistics and data analysis techniques
- Proficiency in data visualization and reporting tools
- Ability to interpret and explain complex data sets
- Expertise in statistical software packages such as SAS, SPSS, R, and Python
- Knowledge of database management systems
- Excellent problem-solving, communication, and organizational skills
- Ability to work independently and manage multiple projects simultaneously
- Ability to effectively collaborate with teams
- Attention to detail and accuracy in data-driven decisions
- Understanding of the ethical implications of data analysis
Statistical analysis is a crucial skill for any data analyst. It enables them to analyze large amounts of data and draw meaningful conclusions from it. Statistical analysis involves the use of techniques such as descriptive statistics, regression analysis, sampling and hypothesis testing.
This skill helps analysts to identify correlations between variables, identify trends, and draw valid conclusions. Statistical analysis is also used to make predictions about future events and develop strategies to address potential problems. With the right tools, any data analyst can turn large amounts of data into useful insights.
By using statistical analysis, analysts can make informed decisions that are backed up by evidence. This can be invaluable in the decision-making process within organizations, allowing them to make informed decisions that maximize resources, minimize risk, and increase efficiency.
Econometrician, Cost Analyst, and Quantitative Analyst are related jobs you may like.
Frequent Interview Questions
- What experience do you have with analyzing and interpreting data?
- How do you ensure accuracy and quality when you analyze data?
- What methods do you use to identify trends and patterns in data?
- How do you think about gathering and organizing data for analysis?
- What experience do you have with statistical software programs such as SAS, SPSS, or R?
- How do you stay informed on new developments in the field of statistical analysis?
- Describe a project you worked on where you had to use statistical analysis.
- What challenges have you faced when working with large datasets?
- How do you go about developing meaningful insights from data?
- What techniques do you use to present data in a meaningful way to non-technical stakeholders?
Common Tools in Industry
- R Programming. R is a programming language and software environment for statistical computing, data analysis, and graphical visualization. It is widely used among statisticians and data miners for developing statistical software and data analysis. (e. g. IBM SPSS)
- SAS. SAS is a powerful statistical software suite used by researchers, analysts, and data scientists for data management, predictive analytics, and advanced analytics. It provides an extensive library of statistical procedures and graphical functions for analyzing and presenting data. (e. g. SAS Enterprise Miner)
- SPSS. SPSS is an IBM software package used for statistical analysis. It includes a wide range of tools for data management, data mining, data visualization, and statistical analysis. (e. g. IBM SPSS Modeler)
- MATLAB. MATLAB is a programming language and environment for numerical computing and visualization. It includes a wide range of tools for scientific computing, data analysis, machine learning, and image processing. (e. g. MATLAB Deep Learning Toolbox)
- Tableau. Tableau is a data visualization tool used to quickly create interactive visualizations and dashboards. It can connect to a variety of data sources, including databases, spreadsheets, and big data warehouses. (e. g. Tableau Desktop)
Professional Organizations to Know
- American Statistical Association
- International Association for Statistical Education
- Royal Statistical Society
- International Biometric Society
- Institute of Mathematical Statistics
- International Statistical Institute
- International Machine Learning Society
- Data Science Association
- Analytics Society of INFORMS
- Big Data Analytics Association
We also have Economic Policy Analyst, Environmental Economist, and Research Associate jobs reports.
Common Important Terms
- Data Mining. The process of extracting useful information from large sets of data.
- Data Analysis. The process of analyzing data sets in order to make informed decisions.
- Regression Analysis. A statistical technique used to identify relationships between dependent and independent variables.
- Hypothesis Testing. A process used to test the validity of a hypothesis by using statistical data.
- Descriptive Statistics. A set of techniques used to summarize and describe data.
- Inferential Statistics. A set of techniques used to make conclusions about a population based on a sample of that population.
- Machine Learning. A type of artificial intelligence that enables computers to learn from data without being explicitly programmed.
- Time Series Analysis. A method used to analyze changes in data over time.
- Multivariate Analysis. A type of statistical analysis used to examine how multiple variables interact with each other.
- Cluster Analysis. A technique used to group data points that share similar characteristics.
Frequently Asked Questions
What is a typical job duty of a Statistical Analyst?
A typical job duty of a Statistical Analyst is to analyze data using statistical techniques and generate reports to inform decision-making.
What qualifications are required to become a Statistical Analyst?
Most employers require a minimum of a bachelors degree in a field such as statistics, mathematics, economics, or computer science for the position of Statistical Analyst. Knowledge of programming languages such as R, Python, or SAS is also beneficial.
What are the job prospects for a Statistical Analyst?
The job outlook for a Statistical Analyst is very good, with the U.S. Bureau of Labor Statistics predicting that the number of jobs in this field will grow by 20% between 2018 and 2028.
How much does a Statistical Analyst typically earn?
The median salary for a Statistical Analyst is around $82,000 per year, according to PayScale.com.
What type of environment does a Statistical Analyst usually work in?
Statistical Analysts usually work in office settings and often interact with other professionals such as data scientists, business analysts, and software engineers.
What are jobs related with Statistical Analyst?
- Labor Economist
- Industrial Economist
- Monetary Economist
- Economic Researcher
- Market Economist
- Financial Risk Manager
- Research Economist
- Budget Analyst
- Commodity Economist
- Data Scientist
Web Resources
- Statistical analyst Career and Professional Development career.asu.edu
- How to Become a Statistical Data Analyst | Maryville online.maryville.edu
- Statistical Analysis | Libraries & Academic Innovation - Gelman library.gwu.edu
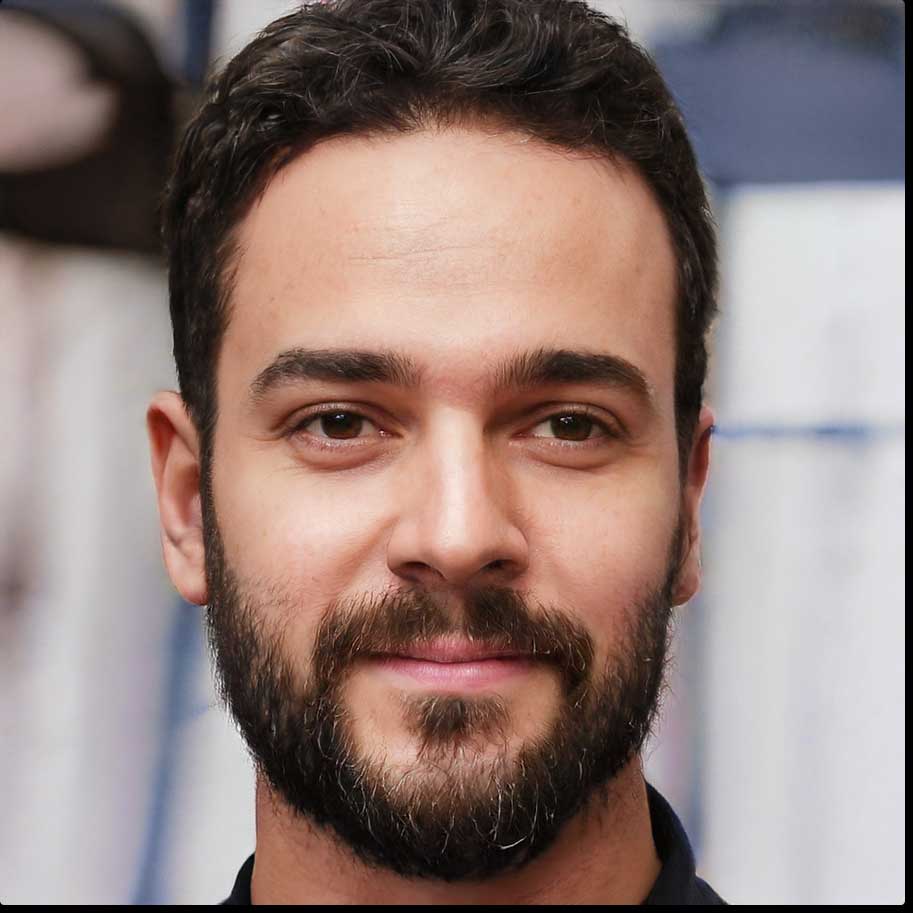