How to Be Exploratory Analyst - Job Description, Skills, and Interview Questions
The use of technology in the workplace has had a significant effect on business operations. Technology has made it easier for businesses to automate many processes, resulting in a decrease in labor costs, increased efficiency, and improved customer satisfaction. technology has enabled businesses to utilize big data analytics, which provides insights into customer behavior and market trends.
This has allowed companies to identify opportunities for growth, develop new products and services, and gain competitive advantages. embracing technology has helped businesses increase their profitability and expand into new markets.
Steps How to Become
- Obtain an undergraduate degree in a related field such as mathematics, computer science, economics, statistics, or business. Many entry-level positions require a bachelors degree.
- Gain experience working with data analysis tools such as SAS, SPSS, and R. Many employers require proficiency in these programs.
- Develop strong problem-solving skills. Exploratory analysts must be able to ask the right questions and interpret data quickly and accurately.
- Take courses in data mining, machine learning, and predictive analytics to gain a deeper understanding of the process.
- Consider obtaining a masters degree in data science or analytics to increase your chances of landing a job as an exploratory analyst.
- Network with other professionals in the field to stay up to date on trends and technologies.
- Stay current on the latest developments in exploratory analysis and industry trends.
- Develop a portfolio of projects to demonstrate your skills and expertise.
The ability to become a skilled and capable Exploratory Analyst requires a combination of technical, analytical, and communication skills. Technical skills involve the ability to use software tools to understand and analyze data, such as using SQL and Microsoft Excel. Analytical skills involve the ability to interpret data, draw logical conclusions, and create reports.
Good communication skills are also essential, as Exploratory Analysts need to be able to explain their data analysis findings to key stakeholders in an effective and understandable manner. By mastering these skills and combining them with a passion for data analysis, one can become a successful Exploratory Analyst.
You may want to check Market Explorer, Exploratory Scientist, and Geographic Explorer for alternative.
Job Description
- Business Intelligence Analyst
- Data Scientist
- Financial Analyst
- Data Analyst
- Database Administrator
- Machine Learning Engineer
- Research Analyst
- Market Research Analyst
- Exploratory Analyst
- Statistical Analyst
Skills and Competencies to Have
- Analytical Thinking: The ability to think logically and objectively to solve problems and draw meaningful conclusions.
- Data Analysis: The ability to examine data sets and draw meaningful insights from them.
- Visualization and Presentation: The ability to create effective visualizations of data and present findings to stakeholders.
- Technical Skills: Proficiency in data analysis software and programming languages such as SQL, Python, and R.
- Communication Skills: The ability to communicate complex information to diverse audiences in a clear and concise manner.
- Business Acumen: An understanding of the business context and implications of data-driven decisions.
- Problem-solving Skills: The ability to identify problems and develop creative solutions.
- Research Skills: The ability to locate and organize relevant data from various sources.
- Statistical Knowledge: An understanding of statistical concepts and techniques for data analysis.
- Attention to Detail: The ability to identify discrepancies and inconsistencies in data sets.
Exploratory analysis is an essential skill for data analysts, as it allows them to gain a better understanding of the datasets they are working with. By exploring the data, analysts can identify relationships and trends between different entities, and can assess the impact of certain variables on the results they are trying to uncover. exploratory analysis helps analysts to develop hypotheses and test them, which is key to developing an understanding of the underlying mechanisms behind the data.
Through this process, analysts can gain valuable insights that can inform their decision-making and help to identify opportunities that can be further explored. having a strong grasp of exploratory analysis is an invaluable asset for any analyst, as it allows them to make sense of complex datasets and uncover potential insights.
User Experience Explorer, Business Explorer, and System Explorer are related jobs you may like.
Frequent Interview Questions
- How have your analytical and problem-solving skills helped you in the past?
- Describe an exploratory analysis project that you completed and the outcome.
- What experience do you have using various data sources such as databases, spreadsheets and statistical packages?
- How do you stay up to date with the latest developments in exploratory data analysis?
- What techniques do you use to visualize data and uncover patterns and trends?
- How do you identify gaps in data or assumptions when conducting an exploratory analysis?
- What challenges have you faced while exploring large datasets?
- How do you decide which exploratory analysis techniques are the most appropriate for a given dataset?
- Describe your process for understanding the business context and requirements prior to beginning an exploratory analysis.
- How do you ensure the accuracy of your findings during an exploratory analysis?
Common Tools in Industry
- Tableau. Tableau is a data visualization tool used to create interactive charts and graphs. (e. g. Clustering algorithms to find relationships in data)
- SAS. SAS is a statistical analysis software package used to analyze large datasets. (e. g. Regression techniques to identify trends in data)
- R. R is an open source programming language for statistical computing and graphics. (e. g. Machine learning algorithms to predict future values)
- Python. Python is a high-level programming language used for automating data analysis processes. (e. g. Automated data cleaning scripts)
- Excel. Excel is a spreadsheet application used to analyze and visualize data. (e. g. Pivot tables to summarize data)
- SQL. SQL is a language used to query databases and retrieve data from them. (e. g. Joins to combine multiple datasets)
Professional Organizations to Know
- American Statistical Association
- Institute for Operations Research and the Management Sciences
- International Institute of Business Analysis
- Association for Computing Machinery
- Association for Information Systems
- International Association for Intelligence Education
- The Institute of Management Consultants USA
- Association of Data Scientists
- International Institute of Business Analysis
- International Association of Business Analysts
We also have Exploratory Data Analyst, Exploratory Marketer, and Adventure Explorer jobs reports.
Common Important Terms
- Data Mining. The process of gathering, analyzing and extracting patterns from large datasets.
- Statistical Analysis. The use of mathematical and statistical methods to explore and describe data.
- Predictive Modeling. Using data to build models that can predict future outcomes.
- Machine Learning. A subfield of artificial intelligence that focuses on developing algorithms and systems that can learn from and adapt to data without explicit programming.
- Data Visualization. The use of graphical displays to communicate and explore data.
- Data Warehousing. The process of collecting, organizing, and storing large amounts of data for analysis.
- Data Wrangling. The process of cleaning, transforming, and integrating data for analysis.
- Business Intelligence. The use of data to inform decision-making and solve business problems.
Frequently Asked Questions
What is Exploratory Analysis?
Exploratory Analysis is an approach to data analysis that involves exploring and uncovering patterns and relationships in data. It is a form of data visualization and statistical analysis used to identify meaningful insights from large datasets.
What techniques are used for Exploratory Analysis?
Common techniques for Exploratory Analysis include data visualization techniques such as scatter plots, heatmaps, bar charts, and histograms. Additionally, statistical methods such as hypothesis testing, correlation analysis, and regression can be used.
What are the benefits of Exploratory Analysis?
Exploratory Analysis can be used to uncover meaningful insights from data that may otherwise be overlooked. It also allows for quicker analysis and interpretation of complex datasets, and can reveal relationships between different variables.
What software is used for Exploratory Analysis?
There are many software packages available for Exploratory Analysis, including R, Python, Tableau, SAS, and SPSS.
How long does Exploratory Analysis take?
The time needed for Exploratory Analysis will vary depending on the size of the dataset and the complexity of the analysis. Generally speaking, it can take anywhere from a few hours to several days or weeks.
What are jobs related with Exploratory Analyst?
- Industrial Explorer
- Space Explorer
- Exploratory Historian
- Exploratory Software Engineer
- Exploratory Writer
- Wildlife Explorer
- Prospecting Explorer
- Research Explorer
- Exploratory Designer
- Financial Explorer
Web Resources
- Exploratory Factor Analysis | Columbia Public Health www.publichealth.columbia.edu
- Exploratory Analysis - 4 | STAT 504 - PennState: online.stat.psu.edu
- Exploratory Data Analysis Overview - Data Science Discovery discovery.cs.illinois.edu
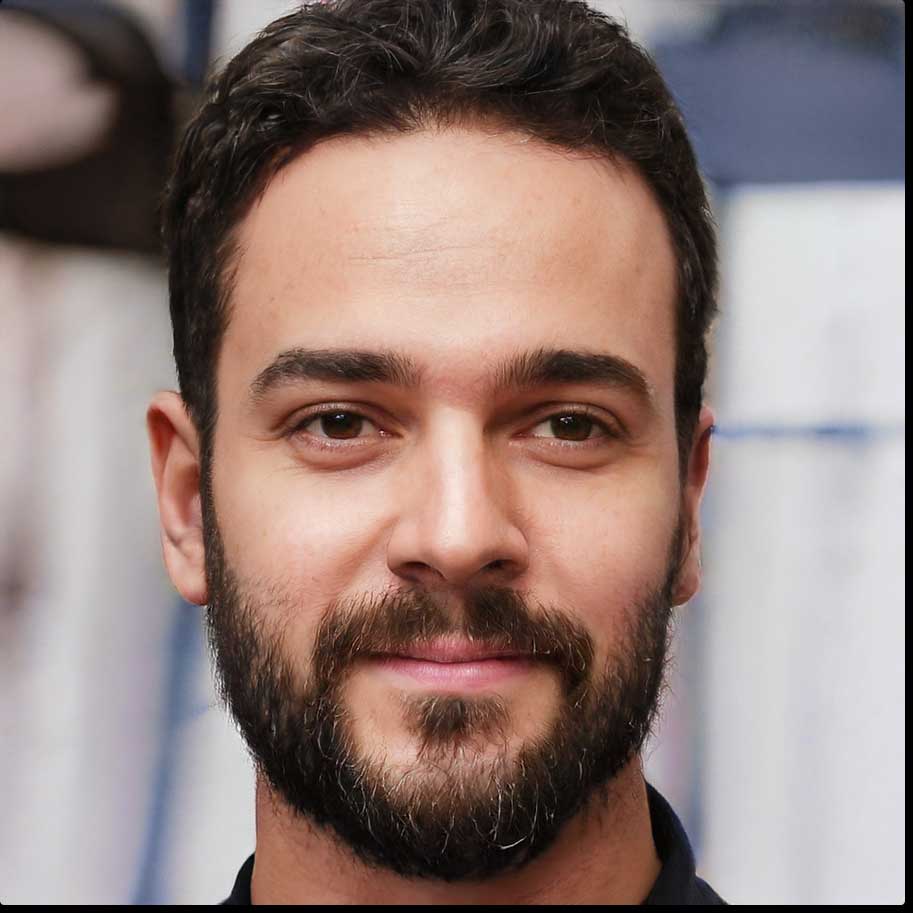