How to Be Data Quality Analyst - Job Description, Skills, and Interview Questions
Poor data quality can have a serious impact on a business. Bad data can lead to incorrect decision-making, higher costs, and decreased customer satisfaction. When data is inaccurate, companies may fail to identify key trends and insights that could help them stay competitive.
poor data quality can result in lost time and resources spent on manual processes to correct inaccuracies. this can mean missed opportunities, as well as reduced profitability and decreased customer loyalty. To address this issue, companies need to prioritize data quality assurance and invest in technologies such as data cleansing and automation tools.
Steps How to Become
- Obtain a Bachelors Degree. To become a Data Quality Analyst, you must have a minimum of a bachelors degree in computer science, mathematics, information technology, or a related field.
- Gain Experience. You can gain experience for a career as a Data Quality Analyst by working in a data-oriented role. This could include roles such as a Data Analyst, Database Administrator, or Business Intelligence Analyst.
- Develop Your Skillset. To become a Data Quality Analyst, you should develop your skillset in areas such as data management, data analysis, data modeling, data cleansing, and data governance.
- Obtain Certifications. Obtaining certifications related to data quality can be beneficial for a career as a Data Quality Analyst. This could include certifications such as Certified Analytics Professional (CAP), Certified Data Management Professional (CDMP), or Certified Data Quality Professional (CDQP).
- Pursue a Masters Degree. Pursuing a masters degree in data science or analytics can be beneficial for your career as a Data Quality Analyst.
- Network. Networking is essential for a career as a Data Quality Analyst. You can join professional organizations such as the Data Quality Association or the International Institute of Analytics to network with other professionals in the field.
Data quality analysts play a vital role in ensuring the accuracy and reliability of data. By utilizing sophisticated software and analysis tools, they are able to identify and address any discrepancies or inaccuracies in the data. This helps to ensure that data is trustworthy and can be used for decision-making processes.
Data quality analysts must be highly knowledgeable and skilled in data analysis, as well as have excellent communication and problem-solving skills. They must also be able to work independently and collaboratively as part of a team. With these qualities, data quality analysts can help organizations make sense of their data, so they can make informed decisions and gain a competitive edge.
You may want to check Data Architect, Data Integration Manager, and Data Migration Specialist for alternative.
Job Description
- Develop and document data quality processes and procedures.
- Monitor data for accuracy, completeness, and conformance to established standards.
- Analyze data profiling and trending to identify potential data quality issues.
- Create data quality dashboards and reports.
- Engage with stakeholders to review and discuss data quality findings.
- Design and execute data quality tests.
- Develop data cleansing and transformation processes.
- Communicate data quality issues, resolutions, and best practices to stakeholders.
- Develop data quality metrics to measure the accuracy and integrity of data.
- Collaborate with IT to develop and implement data quality solutions and tools.
Skills and Competencies to Have
- Analytical and problem-solving skills
- Knowledge of database systems and data management practices
- Knowledge of data mining, analytics, and statistics
- Knowledge of data visualization techniques
- Understanding of quality assurance best practices
- Ability to create reports, dashboards, and other data-driven documents
- Excellent communication and interpersonal skills
- Attention to detail and accuracy
- Ability to interpret complex data sets
- Ability to collaborate with stakeholders and other data professionals
Data Quality Analysts are responsible for ensuring that data is accurately collected, stored, and used. This requires an extensive knowledge of data collection and analysis tools, as well as the ability to detect patterns and identify trends. The most important skill for a Data Quality Analyst is their ability to identify and solve problems proactively.
By understanding the source of data errors and developing effective solutions, Data Quality Analysts can prevent costly mistakes before they occur. Having a strong analytical mindset and excellent organizational skills are also essential for this role, as well as the ability to effectively communicate findings with stakeholders. These skills combined allow Data Quality Analysts to ensure data accuracy and reliability, which in turn leads to better business decisions and more efficient processes.
Data Analyst Manager, Data Integration Specialist, and Data Analyst Intern are related jobs you may like.
Frequent Interview Questions
- What experience do you have with data quality initiatives?
- What methods do you use to ensure data accuracy?
- How do you identify and resolve data discrepancies?
- How do you detect and prevent data duplication?
- How do you audit data standards and processes?
- How do you ensure data integrity?
- Describe a data quality project you have worked on previously.
- How do you stay up to date on the latest data quality trends?
- What strategies do you use to maintain data quality over time?
- What challenges have you faced when implementing data quality initiatives?
Common Tools in Industry
- Trifacta. Trifacta is a data wrangling technology that helps organizations clean and prepare large datasets for analysis. (Example: Trifacta can be used to uncover patterns in large datasets and normalize different data sources into a consistent format. )
- Microsoft Power BI. Microsoft Power BI is a business intelligence and analytics platform that helps businesses collect, analyze, and visualize data. (Example: Power BI can be used to create interactive dashboards, generate reports, and perform data analysis. )
- Tableau. Tableau is a data visualisation platform that helps organisations turn raw data into actionable insights. (Example: Tableau can be used to create interactive dashboards and visualisations that help identify trends and identify areas of improvement. )
- RapideData. RapideData is a data profiling and cleansing tool that helps organisations analyse their data and identify issues quickly. (Example: RapideData can be used to detect inconsistencies in data sources and quickly cleanse data to ensure accuracy. )
- Alteryx. Alteryx is a self-service analytics platform that helps organisations streamline their analytics processes. (Example: Alteryx can be used to quickly build workflows for data preparation, blending, and advanced analytics analysis. )
Professional Organizations to Know
- Data Management Association International (DAMA)
- Association for Computing Machinery (ACM)
- Institute of Electrical and Electronics Engineers (IEEE)
- American Statistical Association (ASA)
- Predictive Analytics World (PAW)
- International Institute for Analytics (IIA)
- American Health Information Management Association (AHIMA)
- Data Science Association (DSA)
- International Association for Information and Data Quality (IAIDQ)
- Strata Conference (O'Reilly)
We also have Data Governance Manager, Data Modeler, and Data Warehouse Manager jobs reports.
Common Important Terms
- Data Governance. The overall management of the availability, usability, integrity, and security of data used in an organization.
- Data Profiling. Examining the data to identify patterns, statistics, and other characteristics of the data set.
- Data Integrity. Ensuring that data is complete, accurate and consistent across systems, applications and databases.
- Data Modeling. The process of creating a logical representation of the data within an organization and its associated relationships.
- ETL (Extract Transform Load). The process of extracting data from various sources, transforming it into a format suitable for analysis and loading it into a database or other storage medium.
- Data Mining. The process of extracting patterns and correlations from large sets of data.
- Data Cleansing. The process of detecting and correcting (or removing) data that is incorrect, incomplete, or duplicated in a database.
- Data Quality Assurance. The process of ensuring that data is accurate, complete, and timely by applying a set of controls and tests to the data.
- Business Intelligence. The process of collecting and analyzing data to gain insight into how an organization can improve its operations.
Frequently Asked Questions
What is the primary goal of a Data Quality Analyst?
The primary goal of a Data Quality Analyst is to ensure the accuracy, completeness, consistency and timeliness of data in an organization's systems.
What skills are essential for a Data Quality Analyst?
Essential skills for a Data Quality Analyst include data analysis, problem solving, critical thinking, data mining, data modeling, and knowledge of data quality tools and techniques.
What types of data does a Data Quality Analyst typically analyze?
A Data Quality Analyst typically analyzes structured and unstructured data from sources such as databases, spreadsheets, web applications and APIs.
What is the job outlook for a Data Quality Analyst?
The job outlook for a Data Quality Analyst is expected to be strong, with the US Bureau of Labor Statistics projecting a 10% growth in employment in the field between 2019 and 2029.
What is the average salary of a Data Quality Analyst?
The average salary of a Data Quality Analyst in the United States is $68,532 per year.
What are jobs related with Data Quality Analyst?
- Data Administrator
- Data Solutions Architect
- Data Science Consultant
- Data Protection Officer
- Data Steward
Web Resources
- Data Quality Analyst Career Center | University of Southern ¦ careers.usc.edu
- Data Quality Analyst | NC State Online and Distance Education online-distance.ncsu.edu
- How to Become a Quality Analyst? | Leverage Edu leverageedu.com
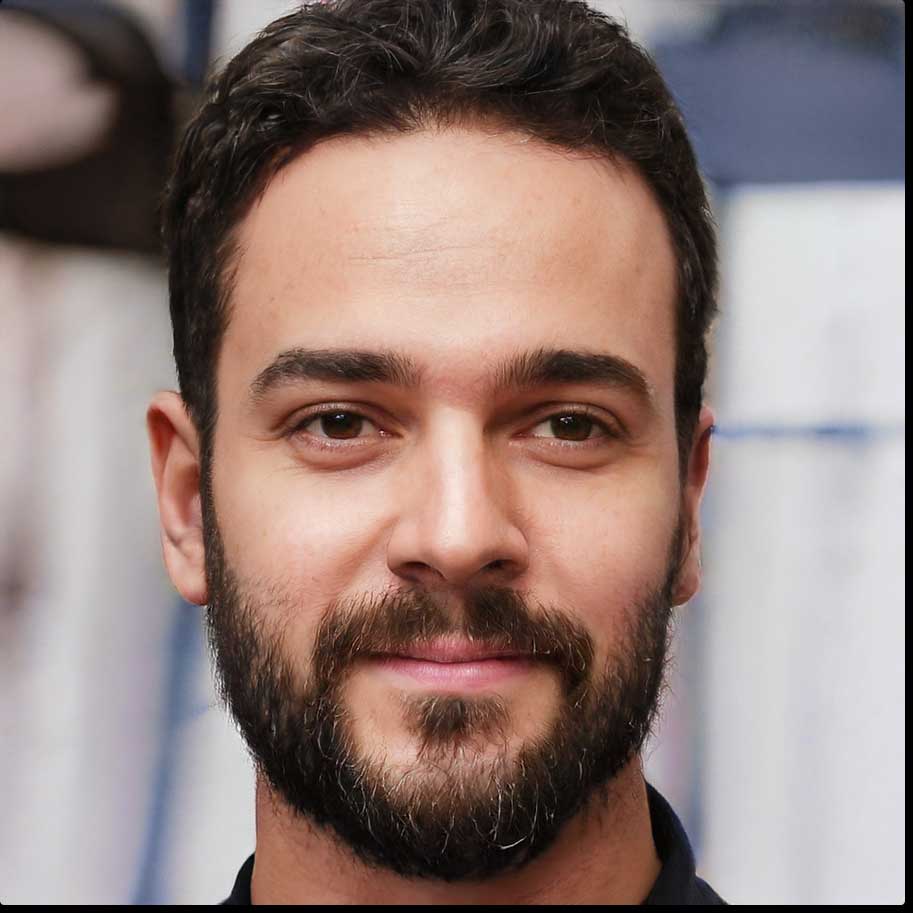